Novel Machine Learning Technique May Help ID Tremor Specific to MS
Program over 90% accurate in differentiating tremor from other diseases
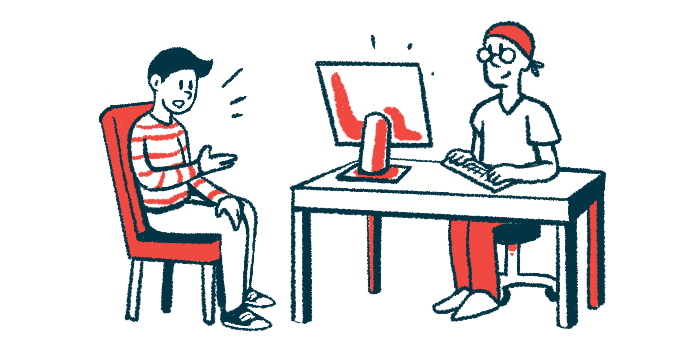
A new machine learning strategy was able to differentiate tremor specific to multiple sclerosis (MS) from tremor related to other diseases, with more than 90% accuracy, according to a new study.
“The proposed method, with high classification accuracy and strong correlations of [tremor] features to clinical outcomes, has clearly demonstrated the potential to complement the existing tremor-diagnostic approach in MS patients,” the researchers wrote.
The study, “Machine learning aided classification of tremor in multiple sclerosis,” was published in eBioMedicine.
Tremor, or uncontrolled shaking, can occur as a symptom of MS, usually associated with damage to part of the brain called the cerebellum. In fact, according to the researchers, about half of MS patients experience this symptom.
However, other conditions related to cerebellar damage, including Parkinson’s disease and essential tremor (ET), also can cause such shaking symptoms.
In all of these conditions, the tremor may appear similar, which can make it hard for clinicians to determine the correct diagnosis for some patients. That can lead to delays in starting treatment for those individuals who are ultimately diagnosed with MS.
New technique tested on patient data
Now, an international team of researchers conducted a machine learning analysis aiming to differentiate between these different types of tremors.
The analysis included data from 40 people with MS, 39 with Parkinson’s, and 41 with ET. In addition to clinical data for each patient, the analysis included accelerometer-based measures of the tremor movements, as well as measures of the electrical activity in patients’ muscles. Such an electrical activity assessment is called an electromyogram, abbreviated EMG.
Like other machine learning approaches, this analysis basically involved feeding data into a computer, and then having the computer make sense of the data based on certain mathematical rules. The researchers “trained” the algorithm using data from half of the patients, and then tested the program on the remaining data.
This analysis used a particular statistical framework called Soft Decision Wavelet Decomposition, or SDWD, to help make sense of the data, the researchers noted. The technique is especially well-suited to analyses of data in which the strength of a signal (e.g., tremor intensity) is constantly changing, according to the team.
“The advantage of SDWD over conventional [statistical] techniques is better discrimination efficiency, particularly for the tremors where frequencies overlap,” they wrote.
Results showed that this analytical method could differentiate MS tremors from ET or Parkinson’s with an accuracy of up to 91.67% based on accelerometer data. Similar accuracies were possible with the analysis of EMG data.
As MS tremor more often resembles that in ET, the team conducted a similar analysis that excluded Parkinson’s patients. While the accuracy of the algorithm was slightly reduced, owing to the greater similarities between the groups, it was still close to 80% based on accelerometer and EMG data.
Additional analyses showed that the metrics the computer used to make these classifications, called “discriminators,” were significantly correlated with clinical measures of tremor severity in the patients.
“The frequency discriminators were excellent predictors of the clinical scores justifying the relevance of the parameters. … These discriminators could complement the other diagnostic criteria presently used in the clinics,” the researchers concluded.
According to the team, the use of this machine learning approach could be a helpful tool in better differentiating MS tremor from tremor in other diseases.
“This proposed technique not only facilitates a method for clinical diagnostic crucial for the timely clinical intervention to slow the disease progression but also provides an insight in the neurobiology of the MS tremor and [its] distinction from [Parkinson’s] and ET,” they noted.