AI model is able to predict MS risk years before disease onset
Data used in model came from more than 3,000 people with or without MS
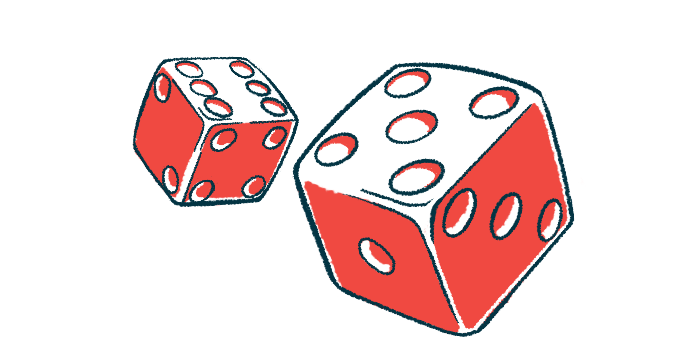
A new artificial intelligence (AI) algorithm could help identify people at risk of developing multiple sclerosis (MS).
“Our model’s performance suggests that AI-based prediction models could identify the risk for multiple sclerosis years before neurological symptoms appear,” Raj Gopalan, MD, a scientist at Siemens Healthineers, Tarrytown, New York, said in a press release.
Gopalan, who led the research, and his colleagues presented the findings at the 2023 American Association for Clinical Chemistry (AACC) Annual Scientific Meeting and Clinical Lab Expo this month in Anaheim, California.
In MS, inflammation in the brain and spinal cord causes damage to nerve fibers. This damage interferes with the nerves’ ability to send electrical signals, causing neurological dysfunction that gives rise to the disease’s symptoms.
Exactly what causes MS to develop remains poorly understood. Many risk factors have been identified, including a history of infection with the Epstein-Barr virus, genetic factors, smoking, age, sex, and even where a person lives. Predicting the risk of developing MS for any individual person remains a difficult undertaking, leading the researchers to use machine learning to try it. In simplest terms, this involves taking a large amount of data and feeding it into a computer alongside a set of mathematical rules or algorithms. Using these, the computer can identify patterns within the data, pinpointing particular sets of traits associated with developing MS to “learn” a model for predicting risk.
What’s the utility of predicting MS risk?
This study used a machine learning algorithm known as a random forest model. The data used to generate the model came from more than 3,000 people with or without MS collected up to three years before a diagnosis. Information in the model included age, gender, metabolic data, and blood markers such as the levels of neutrophils (a type of inflammatory immune cell) and red blood cells, which carry oxygen through the bloodstream.
The model “was highly accurate and demonstrated strong predictive power,” according to the press release, which didn’t provide more information. The results “could reveal which patients should be monitored for periodic neurological and cognitive exams when symptoms appear,” said Gopalan, who noted the findings could be combined with imaging and other tests to help guide decisions about treatment.
Detecting contaminated blood
In another study presented at the meeting, researchers used a different machine learning algorithm to detect contamination in blood samples.
Testing blood is used in many aspects of medicine. In MS, blood tests can help monitor disease progression, evaluate the effects of treatment, and monitor for complications and medication side effects, among other things. Blood samples can sometimes be contaminated by other medical fluids, however, especially when collected from a catheter.
The scientists here analyzed more than 9 million patient samples and simulated IV fluid contamination in some samples. The machine learning setup detected up to 10 times more types of contaminating chemicals than current methods and accurately showed sources of contamination that have been hard to catch with established methods.
“While this won’t immediately reduce the number of contaminated tests, it will hopefully substantially reduce the operational and clinical impact of these events when they do happen and provide us with a better quality metric with which we can prioritize areas for improvement initiatives,” said Nicholas Spies, MD, lead researcher on the project at the Washington University School of Medicine, St. Louis.