New Machine Learning Algorithm Could Help in Diagnosing MS Sooner
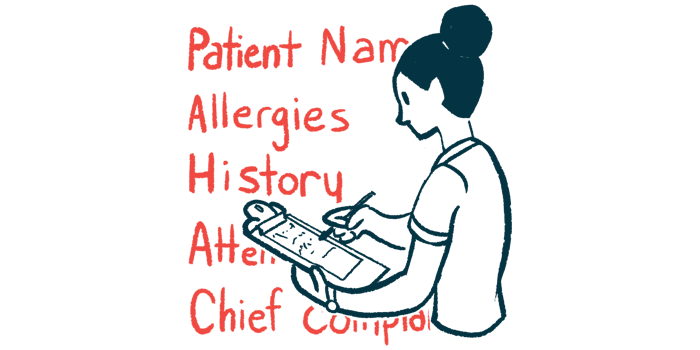
A new machine learning algorithm — designed to analyze healthcare records — could help in diagnosing multiple sclerosis (MS) sooner by identifying patients’ symptoms earlier.
The algorithm, devised by scientists at the University of California San Francisco (UCSF), was described in a study titled “Embedding electronic health records onto a knowledge network recognizes prodromal features of multiple sclerosis and predicts diagnosis,” published in JAMIA.
A growing body of research has shown that many people with MS begin to experience symptoms of the disease years before they get a formal diagnosis. One of the greatest obstacles in diagnosing the neurodegenerative disorder, however, is that many of these early symptoms are mild and not specific to MS.
Since an earlier diagnosis would allow for treatment to start sooner — which is tied to better outcomes later on — there has been a push in research to find new, more accurate ways to identify patients with early MS.
One method that has been explored is the use of machine learning to analyze electronic healthcare records (EHR). Conceptually, machine learning involves giving a computer a set of data, some mathematical rules, and a goal — for example, differentiating between MS or non-MS patients. The computer then generates algorithms aiming to complete the goal.
Although this type of machine learning can be very powerful, it has a notable limitation: by design, most such algorithms don’t take into account what the data actually means, in a biological or clinical sense. Instead, the computer merely is looking for patterns in the data it is given. As a result, there is often a “black box” in the calculation, where it’s impossible to identify the specific factor(s) that the computer is looking at to make its decision.
Now, the UCSF scientists have created an algorithm that aims to get around this issue.
Very simply, the team’s algorithm involves first condensing data from electronic health records into a single biomedical knowledge graph, called a SPOKE. These graphs, in turn, were used to identify health-related signatures, called SPOKEsigs — that, for example, relate to different biological processes or genes.
Ultimately, these SPOKEsigs are what the computer uses to sort people with MS from non-MS cases. Researchers would assess which SPOKEsigs were most important for making the distinction.
Data from 5,752 people in the UCSF healthcare system, all with a confirmed diagnosis of MS, were used by the team in training their algorithm. Specifically, the researchers examined data gathered one to seven years prior to diagnosis. Additional data on more than 2 million people without MS who had visited UCSF between 2011 and 2018 also were included.
The scientists tested the algorithm’s ability to differentiate MS or non-MS by calculating the area under the curve, or AUC — a statistical test that looks at how well a measure can tell the difference between two things. AUC values can range from 0.5, for no ability to tell the difference, to 1 for perfect discrimination.
The AUC for the algorithm or classifier using data from seven years prior to MS diagnosis was 0.76. At one year before MS diagnosis, it was 0.84.
Of note, the algorithm performed better when it included data from all visits rather than information only from primary care physician visits. Moreover, adding the SPOKE data to the electronic health records particularly improved the algorithm’s performance in the three years prior to the diagnosis.
Additional statistical tests indicated that the algorithm’s accuracy will likely improve as additional healthcare data is able to be incorporated.
The investigators noted previous research findings that, in the three years prior to diagnosis, “MS patients have more encounters with psychiatrists and urologists, as well as higher proportions of musculoskeletal, genito-urinary, or hormonal-related prescriptions.”
“These findings hint that underlying biological signals must be present months or even years before diagnosis,” the researchers wrote, adding that “information from these specialist visits could be pivotal in uncovering … differences” between people with and without MS.
Indeed, analyses of the specific SPOKEsigs that were most important for identifying MS were generally in line with the disease’s known biology. For example, many were related to abnormal immune activity or to myelin, the fatty sheath around nerve fibers that is attacked by the immune system in MS.
These results “illustrate how the classifier detected the importance of neurological and immunological processes in MS patients several years before their diagnoses,” the researchers concluded.
“SPOKEsigs represent a new kind of ‘clear box’ explainable predictable models with broad applicability to other chronic medical conditions where early diagnosis can benefit patients,” the team wrote.