Passive Health Tracking Can Predict Changes in MS, Study Says
Apps, fitness trackers collect data to predict depression, symptom severity risk
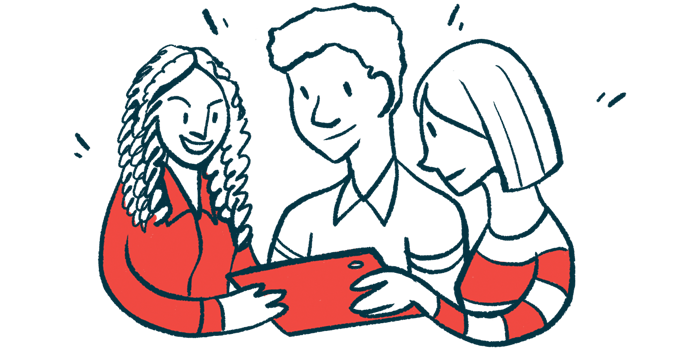
Data passively collected by smartphone apps and fitness trackers can be used to accurately predict the risk of depression, severe fatigue, poor sleep quality, and symptom worsening in people with multiple sclerosis (MS) when in-person health visits are suddenly limited.
These are the findings of a small study that used machine learning to evaluate digital and patient-reported clinical data collected before and during the COVID-19 lockdowns in the U.S. (Machine learning, a form of artificial intelligence, uses algorithms to analyze data, learn from its analyses, and predict outcomes.)
“We were able to capture the change in people’s behaviors and accurately predict clinical outcomes when they are forced to stay at home for prolonged periods,” Mayank Goel, PhD, a professor at Carnegie Mellon University and one of the study’s co-senior authors, said in a university press release.
“Now that we have a working model, we could evaluate who is at risk for worsening mental health or physical health, inform clinical triage decisions, or shape future public health policies,” Goel added.
The study, “Predicting Multiple Sclerosis Outcomes During the COVID-19 Stay-at-home Period: Observational Study Using Passively Sensed Behaviors and Digital Phenotyping,” was published in the Journal of Medical Internet Research Mental Health.
Digital data and patient-reported depression, fatigue and sleep quality
Over the past decade or two, more and more people have begun to use technologies like smartphone apps and fitness trackers in their day-to-day lives. Since these devices gather data by design, they offer an opportunity for clinicians and researchers to better understand the experiences of patients.
Goel’s team, along with colleagues at the University of Pittsburgh and the University of Washington, set out to assess whether data collected from apps and fitness trackers could be used to predict clinically relevant outcomes in MS patients, like worsening fatigue or depression.
They looked at adults with MS who owned a smartphone and were participating in the PROMOTE study (NCT02994121), a 30-year natural history study taking place at the University of Pittsburgh Medical Center.
Participants downloaded a mobile app to capture calls, location, and screen activity data from their smartphones and were given a fitness tracker to track steps, heart rate, and sleep. They also regularly completed standardized patient-reported measures of depression, fatigue, sleep quality, and MS symptom severity.
The original study collected data between November 2019 and January 2021, meaning that some patients had data before and during March 2020, the time that the early COVID-19 pandemic first prompted lockdowns in the U.S.
“It presented us with an exciting opportunity,” Goel said, adding the question that arose was: “If we look at the data points before and during the stay-at-home period, can we identify factors that signal changes in the health of people with MS?”
The analysis included data from 56 patients whose information were continuously collected from Nov. 16, 2019, to May 15, 2020, including the local stay-at-home period. Most were women (86%), white (91%), and non-Hispanic (98%); their average age was 43.5.
Building off of prior research done in first-year college students, the researchers used machine learning algorithms to assess whether the digital data could be used to predict how patient-reported outcomes changed during lockdown.
Notably, many of the assessed outcomes were found to affect each other. For example, fatigue and sleep quality appeared to influence the association between MS severity and depression.
Passive behavior changes in COVID lockdown predict health outcomes
Results showed that the best model, which included data on calls, heart rate, and location, could predict the occurrence of depression during the stay-at-home period with an accuracy of 82.5%. The same types of data yielded the best predictions of severe fatigue (versus mild fatigue) with an accuracy of 75.5%.
In terms of sleep quality, the best model, including data on location and screen time, was able to predict poor sleep quality (versus better sleep quality) with an accuracy of 84%. The best model for predicting high global MS symptom burden had an accuracy of 90%, based on data from calls, heart rate, location, and screen time.
“Our study provides the first real-world evidence of potential clinical utility of passively sensed behavior changes to predict health outcomes during the unique stay-at-home period in a population with a chronic neurological disorder and complex health needs,” the researchers wrote.
They proposed that using this type of data in routine practice could help with patient care, especially during major stressful events, such as pandemics and natural disasters, that could limit in-person visits and considerably alter behaviors.
“Predictive models built using our approach could help patients self-monitor their health when access to … care becomes suddenly limited and could encourage patients (or their caregivers) to actively seek medical attention sooner” should the models show troubling changes, the team wrote.
“Further, our models could help clinicians better monitor at-risk patients and make triage decisions for patients who require prioritization for interventions (eg, medication and counseling), particularly in the setting of suddenly limited health care access and scarce resources,” they added.
The scientists noted, however, that this analysis was limited by the reliance on patient-reported data to assess outcomes, as well as the small number of participants.
“This study paved the way for future replication studies during major stressful events and has implications for future patient self-monitoring and clinician screening for urgent interventions in MS and other complex chronic diseases,” the team concluded.