Combined Data May Predict Person’s Risk of Advancing From CIS to MS
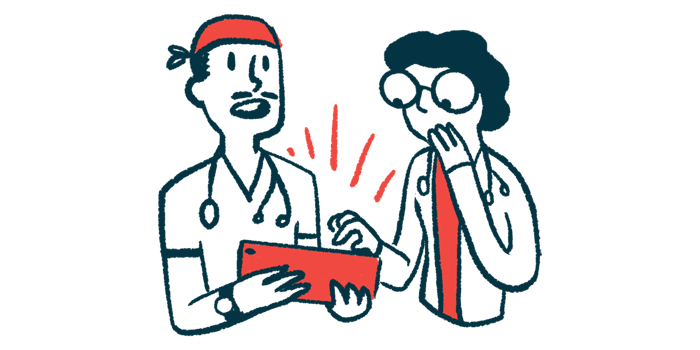
A novel algorithm that combines genetic, environmental, and clinical data could be useful for predicting whether people with a first onset of multiple sclerosis (MS)-like disease — known as clinically isolated syndrome (CIS) — will experience relapses or a worsening of their disease over time.
The algorithm may be particularly useful for selecting patients in clinical trials, its researchers noted.
A team led by scientists at the University of Tasmania in Australia described the algorithm in the paper, “Developing a clinical-environmental-genotypic prognostic index for relapsing-onset multiple sclerosis and clinically isolated syndrome,” published in Brain Communications.
For most patients, MS is characterized by relapses — when symptoms suddenly worsen — followed by remissions, or periods of partial or complete recovery from symptoms. A person with a single instance of an event that looks like an MS relapse is said to have clinically isolated syndrome.
Most people with CIS will eventually have more relapses, which can lead to a formal diagnosis of MS (the “multiple” in “multiple sclerosis” refers to more than one such attack). But some with CIS may go years or decades without experiencing another attack, if they ever do at all.
Figuring out which patients are more or less likely to have additional attacks remains a major challenge for researchers and clinicians. Better methods for making these predictions could result in more effective and personalized treatments, as well as better use of healthcare resources.
The study looked at 253 people (77.5% female) with CIS, defined by a first demyelinating event in the central nervous system (brain and spinal cord), making 2,858 repeated observations over its 10 years. Participants average age was 36.6.
Researchers collected genetic information on nearly 200 genes known to be linked with MS risk, and assessed clinical and environmental factors, ranging from age and sex, to brain lesions, smoking history and sunlight exposure.
By the time the study concluded, 219 participants had more disease features and were diagnosed with MS, while 34 were still classified as CIS.
Using their collected data, researchers constructed a mathematical model to predict the likelihood of a disease relapse, naming it the clinical-environmental-genotypic prognostic index (CEGPI).
Statistical tests showed that, for each unit increase in the CEGPI, there was a roughly 73% higher annual chance of disability worsening, a 68% higher chance of a disease relapse, and a 67% higher chance of either occurring.
These results show that the algorithm “provided robust dynamic estimates for the risk of worsening, relapse, and a combination of these metrics,” the researchers wrote.
Other tests and analyses of the model indicated that, in the short term, environmental and clinical factors played a much bigger part in determining the prognosis (a disease’s likely course) compared with genetic factors. Notably, CEGPI was better at making accurate prognoses than models with only genetic or clinical/environmental data.
“Although the genetic variations provided additional prognostic values for disease time-course prediction, the clinical and environmental components were the major contributors,” the team wrote.
This study’s most important limitation is that CEGPI has not yet been tested on outside data, its researchers said, noting that more study was needed to validate and refine the algorithm.
“Our CEGPI provided reliable information that is relevant for long-term prognostication, but is more applicable as a clinical research tool,” the scientists concluded. “If externally validated, it may be used in risk stratification and selection criteria in clinical trials.”