Assess MS: a New Therapy Assessment Tool Developed by Microsoft – Novartis Collaboration
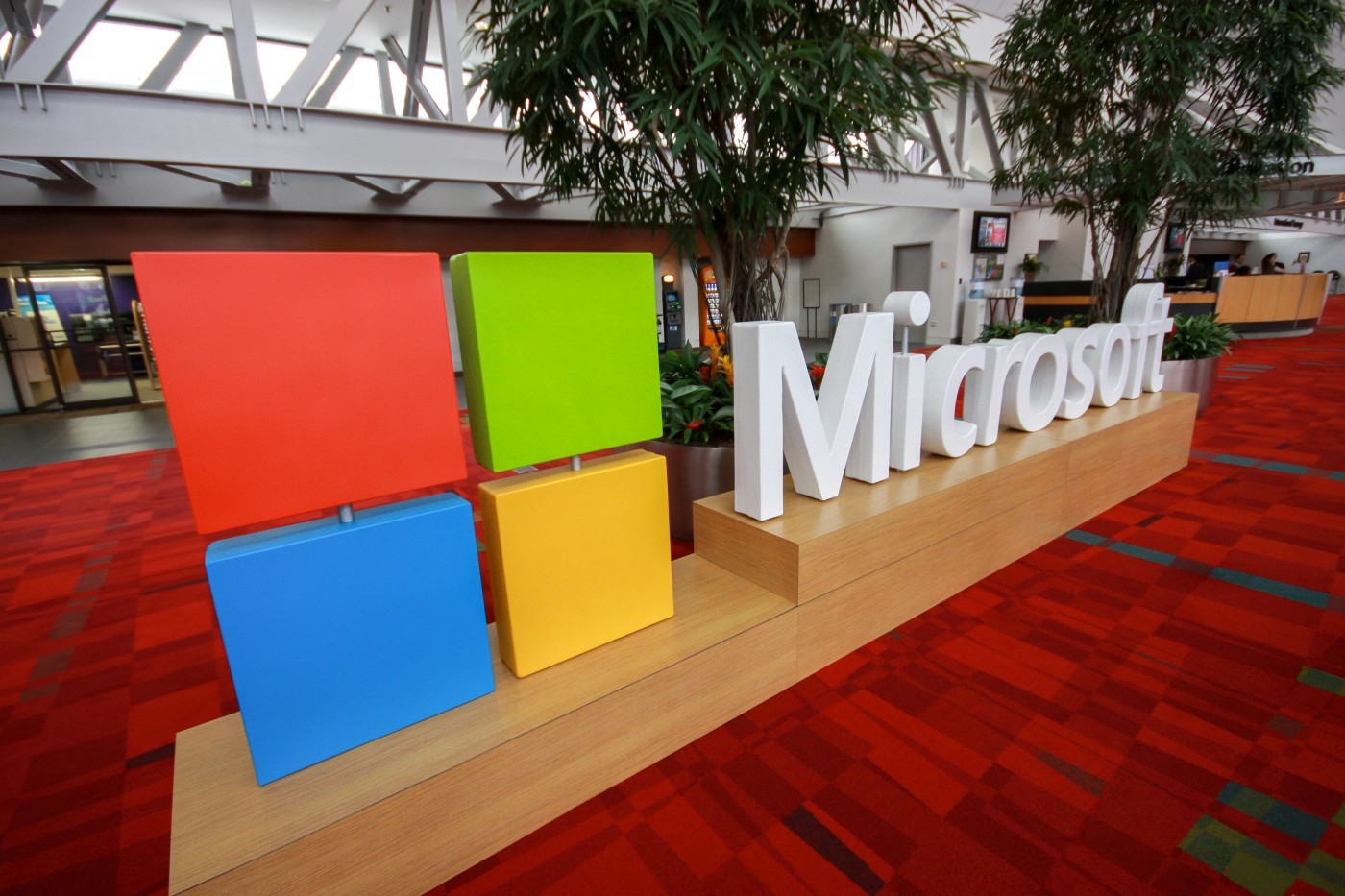
When Microsoft rolled out its original Kinect system for playing Xbox video games in November 2010, the technology caught the eye of giant multinational healthcare company Novartis.
A longstanding research challenge for Novartis has been to devise and design more consistent methods of quantifying whether multiple sclerosis (MS) treatments under development were working. However, they found that making accurate assessments of whether a patient’s MS symptoms were stabilizing or getting worse had proved complicated and elusive.
That difficulty was due in part to the disorder itself being complicated. For example, while MS symptoms progress in some patients with disheartening speed, in others they manifest slowly, sporadically, and over an interval of time that in some cases can stretch over many years.
One attempt to quantify MS progress is the Kurtzke Expanded Disability Status Scale (EDSS) developed by John F. Kurtzke — a method of quantifying disability in MS. The EDSS employs a standard battery of tests, such as asking patients to touch their noses or sit with arms outstretched, enabling doctors to monitor the patient and apply a rating system and more scale to determine how the patient’s symptoms are advancing. The EDSS quantifies disability in eight Functional Systems and allows neurologists to assign a Functional System Score to each of these. The first public EDSS calculator has been developed, which allows standardization of neurostatus scoring.
However, the problem is that doctors are still subjective in making their assessments, so despite worthy efforts to develop standardized MS testing, in the end the evaluation is still subjective. In a recent news release, Microsoft noted, “The researchers found that when a groups of doctors are shown the same patient doing the same movement, some may interpret it as a 1 on the rating scale, while others will say it’s a 2. Even when the same doctor is shown the same movement on two different days, that doctor may give that patient a different rating.”
“One of the most difficult things about MS is the uncertainty of it,” said researcher Cecily Morrison, in the Microsoft release. Morrison has spent the last couple of years at Microsoft’s Cambridge, England, research lab, working on the research project that became Novartis’s idea, called Assess MS.
Morrison describes the project on her Microsoft website as a collaboration among Novartis Pharmaceuticals, three hospital clinics, and the Microsoft Machine Learning and Perception Research Group, for the purpose of designing a system to support clinical assessment of MS using depth-sensing computer vision with the project’s objective to provide a more consistently quantified measure of an MS patient’s motor ability than is currently possible using paper and pencil tools.
Morrison said “the system is specifically designed to work in a clinical setting and to address issues from data capture to data visualization, and that there has been a substantial policy push to get clinical information systems into practice in hope of reducing medical errors and decreasing cost of care. My research focused on how we can design clinical information systems to fit the situational use of data in clinical settings. Studies covered topics from collaborative use of clinical information systems during ward rounds to the extraction of meaningful data for research.”
“The clinicians that we worked with really care about their patients. They really want what’s best for them, and even the best neurologist will admit that when they use these rating scales, it’s pretty coarse-grained,” said Abigail Sellen, a principal researcher at Microsoft Research Cambridge and manager of the interdisciplinary Human Experience & Design (HXD) group, whose focus is on the human perspective in computing. HXD is interested in learning from everyday life to inform the design of systems which are both useful and compelling, and in designing technologies which work in partnership with people. “They know that there’s a lot of variability, even in their own judgments, over time.”
Consequently, the prospect of using the sort of technology used in Microsoft’s Kinect system — computer vision — particularly intrigued Novartis researchers, who figured the use of such a tool would enable a more objective determination of patients who had executed a range of tests, and achieve a higher degree of evaluation consistency that would help doctors more accurately assess the disease’s progress in individual patients, thereby streamlining the process of delivering appropriately matched treatments to these patients.
The researchers’ objective was not removing doctors from the evaluation process, but instead to supplement and enhance their knowledge of MS with a more consistent evaluation of symptoms, analogically similar to tools and methods ophthalmologists use to measure a patient’s declining eyesight.
“What you don’t want to do with these systems is replace the expert. You want to bolster the expert,” Sellen said. “What we’re doing is giving them a set of data that they can then weave into their judgment.”
The news release reports that the Novartis proposal immediately appealed to and excited computer scientists working in Microsoft’s Technology and Research department, noting that researchers at the company have long been at the cutting edge of developments in the computer engineering field of machine learning — a category of artificial intelligence research in which systems progressively improve their performance as they collect and compile more data — an attribute ideally suited to a project like Assess MS, because as the computer vision system captures and records more patient movement, data more consistency can be achieved in assessing the disease’s progression in a particular individual.
Novartis’s challenge presented Microsoft scientists with an opportunity to develop new advances in the machine learning field while speeding access to new treatment options for the estimated more than two million people with MS. But to achieve that objective, it would be necessary to develop entirely new methods of training machine learning systems. “It was clear that this was a very ambitious project,” said Peter Kontschieder, a Microsoft postdoctoral researcher and builder of many of the machine learning algorithms used in the Assess MS project.
Machine learning models require massive amounts of data, such as a collection of images of a certain object. Using a machine learning algorithm, a computer uses those pictures to create a model that recognizes a subject item’s appearance so that when it next encounters an example, even if it isn’t one of the images it was originally shown in the sampling process, the program will be able to identify it.
Thus, the first challenge for scientists working on the Assess MS project was to gather a critical amount of relevant data. In order to accurately assess how a particular MS patient is doing, they needed to devise a way to enable the Microsoft Kinect depth camera, designed as it is to recognize sweeping gestures executed by someone playing a living room video game, to detect more subtle movements such as the sway of a patient’s torso — a requirement that obliged researchers to create entirely new algorithms that would work in the background with Kinect’s depth camera, and would be able to both recognize MS patients’ body components in “a more nuanced way,” thereby yielding more precise representations of how the patient performed in executing the slate of tests.
However, even after creating the necessary algorithms from a blank sheet, the machine learning engineers were still aware that they would be unable to collect an ideal amount of data for the purpose of creating a machine learning model in this context because they were gathering these types of recordings from scratch, and were limited in the ways they could use collected data due to stringent patient privacy restrictions.
Yet another challenge confronting researchers was that unlike basic image recognition — for example, identifying whether a picture is of a tree or a car — the Assess MS project required researchers to get the machine to recognize much more subtly nuanced input data indicating even small progression of MS symptoms.
Overcoming these various difficulties required new techniques for consistent data labeling in order to create a robustly strong model for accurate quantification of results. “We had to think hard about better, more accurate algorithms that would really pick up on the subtle signals that differentiate between an MS patient and a healthy individual,” explained Antonio Criminisi, a principal researcher in machine intelligence and perception at Microsoft’s U.K. lab.
Meanwhile, as Kontschieder, Criminisi, and other machine learning experts were developing the required algorithm innovations, other Microsoft research teams tackled the need to create a system that would be user-friendly for both doctors and patients to operate in real-world settings with a Kinect-based system compact enough to, for example, fit into possibly small or crowded clinical examination rooms with obstacles like chairs, tables, and desks in the way, and a user interface easy for doctors, nurses, and other clinicians to operate.
“We needed to ask, right from the beginning, ‘How do we make it work in the clinics?’” asked Morrison, whose computer science expertise is in human-computer interaction. Morrison noted that a common pitfall in such projects is that researchers typically design tools in laboratory settings, with none of the constraints that will be encountered in a real doctor’s office, then wonder why the technology doesn’t work in practice the way it did in the lab. “It needs to be a collaboration between the patient, the clinician, and the technology,” Morrison said. “What we try to do is strike a balance.”
The Microsoft release notes that the project’s human-computer interaction team discovered that some medical professionals stood in front of the patient to demonstrate movements, thereby blocking the camera’s view, which they addressed by designing a secondary screen that could move behind the camera, subtly guiding clinicians out of the way while still allowing them to monitor the patient. They also created a simple animation model of a human to demonstrate the movement, guiding the patient in the various movements and ensuring their being well-positioned for the camera to detect the movement accurately. They added a way for the clinician to notice if something is obstructing the camera, and equipped the machine with wheels to make it simple to reposition if something gets in the way. “We designed the prototype to help the clinicians help the computer, so that they could get the data they want,” Morrison said.
Microsoft reports that after years of design and algorithm development work, researchers are finally ready to test a proof-of-concept on a small cohort of patients, to confirm that the system can be effective in principle. The next step will be to test Assess MS in practice in order to learn and assess its effectiveness across a broader patient sampling.
In the meantime, the research and development team will continue refining algorithms they apply by measuring patients’ symptoms using new data collected, aiming to enable Novartis and other healthcare companies to use Assess MS to streamline clinical trials for MS treatments, and maybe, eventually, for other neuromuscular diseases, too.
“Novartis is leveraging digital technologies to transform patient care and drug development,” said Vas Narasimhan, global head of development at Novartis Pharmaceuticals. “We are excited about our collaboration with Microsoft Research to develop Assess MS, a more consistent way to measure motor dysfunctions caused by multiple sclerosis, which could lead to the development of better therapies and care for patients.”