$100K Grant Awarded to Study How Patients Are Adhering to Oral DMTs
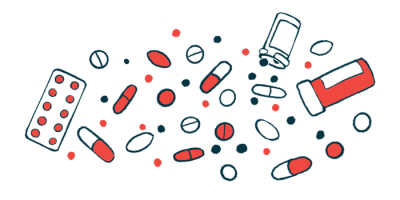
A researcher at the University of Houston has received a $100,000 grant to investigate adherence to oral disease-modifying therapies (DMTs) among people with multiple sclerosis (MS), and correlations between adherence patterns and disease outcomes over time.
The one-year grant from the Agency for Healthcare Research and Quality was awarded to Rajender R. Aparasu, PhD, a professor of pharmaceutical health outcomes and policy at Houston’s College of Pharmacy.
The project will focus on adherence to the first three oral medications for MS: Gilenya (fingolimod), Aubagio (teriflunomide), and Tecfidera (dimethyl fumarate).
“Overall, the aim is to inform clinicians and patients regarding treatment decisions with regard to the use and adherence to oral DMAs [disease-modifying agents], and ultimately to improve health outcomes,” Aparasu said in a university press release.
Oral DMTs are thought to improve adherence to treatment, because they are more convenient than injectable treatments. Yet, real-world data suggest that about 20% of MS patients fail to adhere to these oral medications, and 25% stop using these therapies after one year.
Poor adherence to DMTs and their discontinuation are linked to worse clinical outcomes, higher rates of relapse and disease progression, and greater use of medical resources.
Monitoring adherence to these prescribed medications, and understanding how patterns of adherence impact patients’ outcomes is key to improve care. However, according to Aparasu, current methods for measuring treatment adherence fail to account for changes in adherence over time.
In his research project, Aparasu plans to use a distinct statistical method — called group-based trajectory modeling (GBTM) — to track time-related changes in adherence. With this method, rather than grouping patients by how long they had been prescribed medication, patients are categorized according to their prescription-filling pattern over time.
“Group-based trajectory modeling provides a more accurate picture of adherence patterns over time than more traditional methods of measuring adherence based on refill records or claims in which adherence is assessed as a single value,” Aparasu said.
“With trajectory modeling, patients are classified into different adherent trajectory groups such as complete adherers and slow and rapid discontinuers based on the prescription-filling pattern over time, with each trajectory representing a distinct adherence pattern,” Aparasu added.
After grouping patients according to their adherence pattern, the researcher will determine the clinical outcomes associated with each pattern, including relapse rates requiring hospitalization or a visit to the doctor and a corticosteroid prescription within 30 days.