New Gait Analysis Tool Found to Correctly ID MS Patients in Videos
Tool aims to use walking in real-world setting to tell MS from Parkinson's
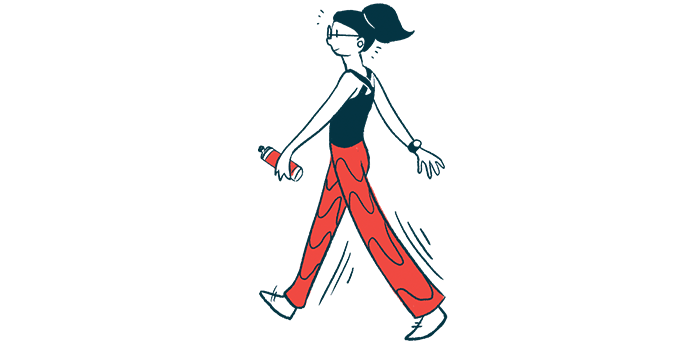
A new tool that analyzes gait, or walking patterns, of people on a treadmill — using video recordings from a simple digital camera — is able to accurately identify those with multiple sclerosis (MS), a study showed.
Scientists said the gait-analysis tool may be a low-cost and easy-to-use way of identifying possible MS patients — and differentiating them from people who may have Parkinson’s disease — using real-world settings.
“To be able to gather gait information using nothing more than a digital camera and have that data assessed online could allow clinicians to do a quick screening that sends to a specialist only those [individuals] deemed likely to have a neurological condition,” the research team, from the University of Illinois, said in a press release.
For those from rural areas who may need to travel for specialized care, or patients with poor access to healthcare services, such machine-learning algorithms could help in getting an MS or Parkinson’s diagnosis sooner, the team noted.
The study, “A Vision-Based Framework for Predicting Multiple Sclerosis and Parkinson’s Disease Gait Dysfunctions – A Deep Learning Approach,” was published in the IEEE Journal of Biomedical and Health Informatics.
People with MS and other neurological disorders, such as Parkinson’s disease, commonly have atypical gait and difficulty walking. While these symptoms are very common, there has been relatively little research that specifically characterizes the differences in gait among people with neurological diseases.
Developing a gait analysis tool
One major reason for the dearth of research in this field is that detailed tests of gait typically require specialized sensors worn across various parts of the body. This equipment is expensive and often requires some expertise to operate correctly, making it difficult to perform gait analysis outside of carefully-controlled laboratory settings.
Now, a Midwestern team of scientists set out to perform gait analysis using video recordings from a simple digital camera. Their study included 33 adults — a majority with neurological disorders, along with healthy people who served as controls.
Among them were 10 adults with MS (seven women and three men) who had a mean age of 66. Nine Parkinson’s patients (six men and three women) with a mean age of 68, also were included in the study. Rounding out the participants were a total of 14 age-, sex-, weight-, and height-matched adults with neither condition. Nine of the controls were women and three men.
All participants underwent a self-paced walking assessment on a treadmill twice: once while just walking, then again while walking and simultaneously talking. Specifically, “talking” involved reciting every other letter of the alphabet in sequence.
This experimental setup was meant to more closely mimic the real-world challenges of walking while performing other potentially mentally distracting tasks, the researchers noted.
“This is a novel study in that we were trying to address the fact that the lab is different from how people behave in the wild,” said Richard Sowers, PhD, one of the study’s authors and a professor at University of Illinois.
“When you’re at home, you’re doing whatever you’re doing, but you’re also thinking, ‘Did I close the garage door? Did I turn the stove off?’ So there’s an added cognitive load,” Sowers said.
The team then used algorithms to identify joints that move as a person walks, and analyzed their movement patterns to extract mathematical representations of various features of gait.
“We looked at the body coordinates for hips, knees, ankles, the big and small toes and the heels,” said Rachneet Kaur, the study’s first author and a graduate student at Illinois.
The researchers then tested whether the collected gait features could be used to reliably identify people with the neurological disorders using machine learning.
Basically, the team fed the data into a computer, and the computer used a prespecified set of mathematical rules to “learn” how to tell the groups apart based on gait features. More than a dozen different well-established machine learning calculation setups were tried for these tests.
The machine learning algorithms were “trained” using gait data from when the participants were walking only. Then, it was tested on gait data from participants walking and talking at the same time.
Specific outcomes varied depending on the specific machine learning parameters, and “no one specific [machine learning] architecture was found to yield the best performance across different features, tasks, and frameworks,” the researchers wrote.
Tool shows accuracy
Nonetheless, results generally showed that these methods could accurately identify MS or Parkinson’s patients based on gait features, with an accuracy up to 75%. This represents “a similar level of performance” as reported in prior gait-focused studies that used specialized worn sensors, the researchers wrote.
“Our system requires only an inexpensive digital camera, and thus can be easily and economically deployed in homes of older adults for a real-time gait analysis with negligible user interaction,” the team wrote.
“This might in turn benefit both patients as well as clinicians to curtail MS and [Parkinson’s] therapy expenses; and further facilitate low-cost and data-driven telemedicine systems for [these patients],” they added.
The low cost and simplicity of the system “should aid in making it a viable point-of-care technology for early detection of gait alterations in real-world settings,” the researchers wrote.
The researchers noted that the study was limited by its small sample size. They also highlighted a need for further work to refine these tools — for example, figuring out how many steps to include for optimal results in the analysis.
Making the tools publicly available will likely take a few years, the scientists noted, though in the meantime they have made the raw code publicly available on GitHub.