New Machine Learning Algorithm May Better Predict Severity in MS
Using genetic data, algorithm can help to choose best treatment
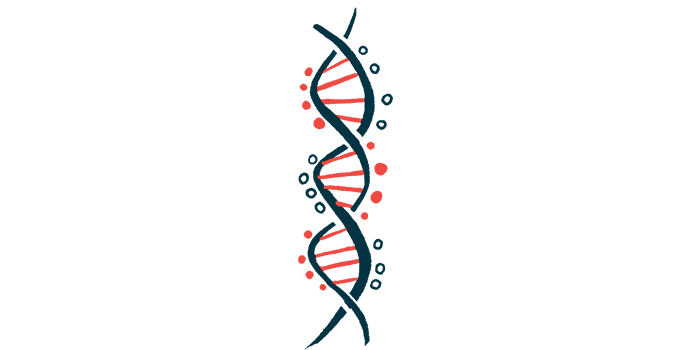
A machine learning algorithm that incorporates genetic data alongside clinical and demographic information may be able to more accurately predict the severity of multiple sclerosis (MS), according to a new study.
“Once independently validated, the machine learning algorithm could enable clinicians to provide patients with more accurate prognostic information, that would then also help to select the most appropriate disease-modifying therapy based on a person’s likely long-term MS outcomes,” Vilija Jokubaitis, PhD, a professor at Monash University, in Australia, and co-author of the study, said in a university press release.
The researchers called the study’s findings “a milestone in our progress towards understanding the clinical heterogeneity of multiple sclerosis outcomes.”
The study, “Not all roads lead to the immune system: the genetic basis of multiple sclerosis severity,” was published in the journal Brain.
Machine learning algorithm ‘reads’ over 60,000 data
A person’s genetic code can have profound impacts on individual health and disease outcomes — many of which are only beginning to be understood. Several genetic variations have been linked with an altered risk of developing MS. But there has been little evidence to suggest that genetics affect disease severity.
To learn more, an international team of researchers conducted an analysis of genetic and clinical data collected from 1,813 people with MS included in the MSBase, an international MS registry.
Notably, all of the patients included the study had relapse-onset disease. In other words, the study did not include people with primary progressive MS, who tend to experience a different disease course.
The researchers’ main analysis did not identify any genetic variants that had a large, or even moderate effect on MS severity.
“Given that no genetic variant associates strongly with disease outcomes, the findings suggest that MS disease outcomes are, to an extent, modifiable with appropriate and early treatment with disease-modifying therapies,” Jokubaitis said.
The scientists did identify a number of variants that had a small effect on severity in the statistical models. Many of these were in genes known to be related to the function of the central nervous system, comprised of the brain and spinal cord. Specifically, these involved synaptic plasticity, or the brain’s ability to “rewire itself” in response to new information, as well as the production of myelin, the fatty sheath around nerve fibers that is damaged in MS.
The team used data on more than 62,000 genetic variants, in addition to demographic and clinical data, to train the machine learning algorithms. In simplistic terms, the data were fed into a computer that used mathematical algorithms to “learn” from the information, identifying factors associated with disease severity.
This algorithm was found to predict disease severity with a high accuracy, of about 84%. The team noted that the algorithm that included genetic data outperformed similar algorithms that only used demographic and clinical data, and had an accuracy of 54%.
“We demonstrate that machine learning using common [genetic] variant clusters, together with clinical variables readily available at diagnosis can improve prognostic capabilities at diagnosis,” the researchers wrote, adding that “with further validation [the machine learning algorithm] has the potential to translate to meaningful clinical practice change.”
The team added that this work represents a milestone towards understanding the clinical variability of relapsing forms of MS.
“The work was a long journey, in fact it was 10 years in the making from writing the first ethics application through to recruiting participants, and performing the analyses,” Jokubaitis said.
“It would not have been possible without our amazing team and collaborators, nurses involved in research and people with MS who generously donated their samples,” she added.