New AI Method Detects Brain Changes in Response to Treatment in RRMS Patients
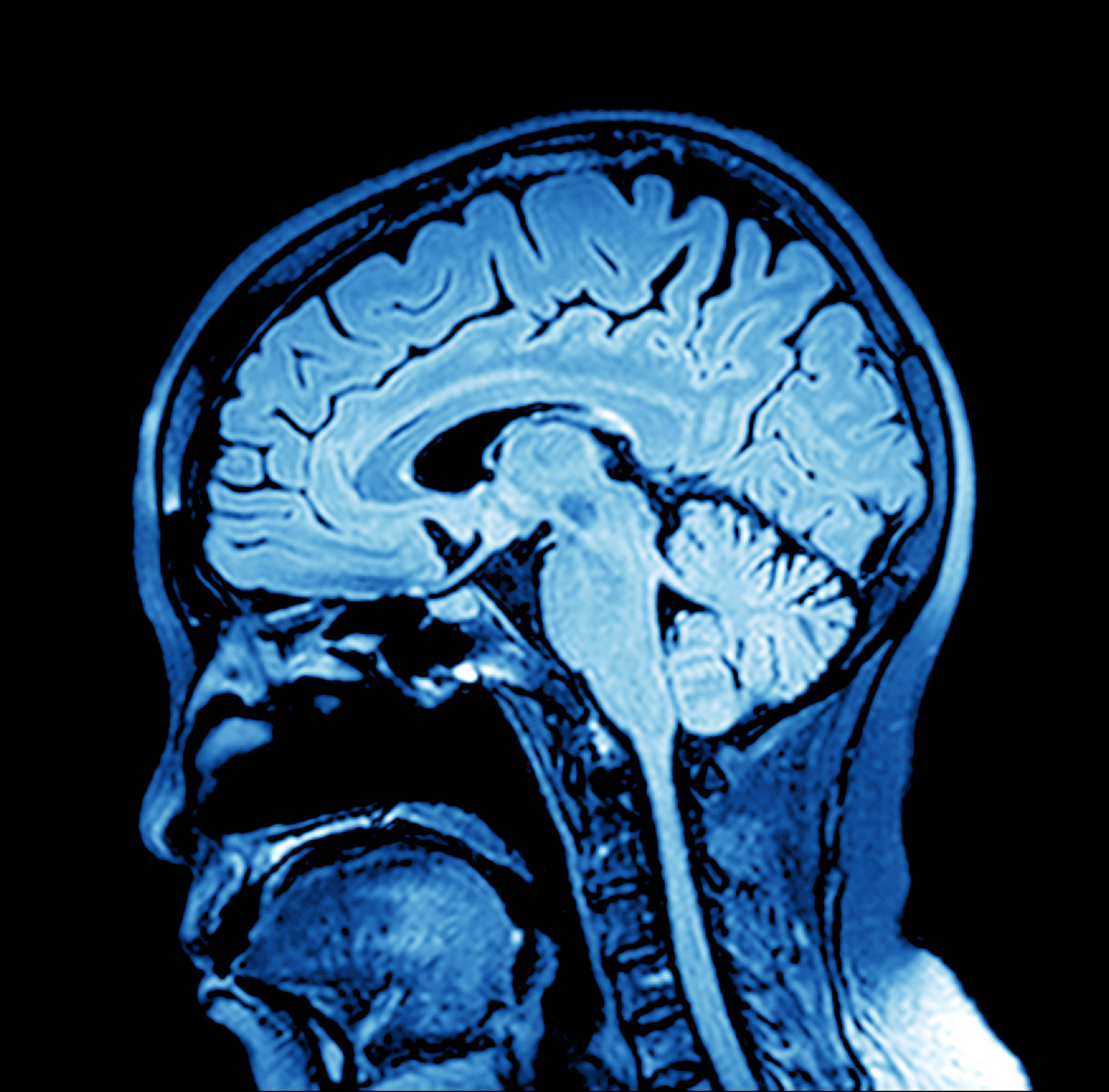
A new artificial intelligence (AI)-based model is better than conventional methods for detecting brain changes in response to treatment with Tysabri (natalizumab) in patients with relapsing-remitting multiple sclerosis (RRMS), a study reports.
The study, “High-dimensional detection of imaging response to treatment in multiple sclerosis,” was published in the journal Nature Digital Medicine.
In patients with multiple sclerosis (MS), brain alterations revealed through imaging techniques such as magnetic resonance imaging (MRI) not only support the diagnosis, but also allow clinicians to predict the course of the disease.
However, in MS, these alterations have a complex distribution and, in most cases, are not easily detected by current radiology-based models.
“This hinders our ability to detect treatment effects, both in the management of individual patients and in interventional trials,” the researchers wrote.
This group, led by researchers from the University College London (UCL) in the U.K., compared the performance of an AI-based model to that of conventional radiology-based analyses for detecting brain changes related to treatment response in a group of RRMS patients.
They included a total of 124 RRMS patients whose brain imaging scans were available before and after starting treatment. Patients were treated with Tysabri, an approved antibody-based therapy manufactured by Biogen.
Using fully automated image analysis, the investigators extracted 144 “image fingerprints” showing brain structures in high detail from patients’ MRI scans obtained before and after treatment. These “image fingerprints” were then used to detect and calculate changes in brain volume and composition over time.
Results showed the best AI-based model surpassed the accuracy (86.5% versus 66.4%), sensitivity (77.7% versus 61.8%), and specificity (77.8% versus 66.8%) of the best conventional model, when it came to detecting brain alterations occurring in response to treatment with Tysabri.
“The method is currently focused on imaging changes only; we are extending the approach to predicting the clinical response to disease-modifying treatment, in terms of cognitive and motor outcomes. I hope that this exciting field of research will lead to an individual prediction of treatment response in multiple sclerosis using AI,” Olga Ciccarelli, PhD, from the UCL Queen Square Institute of Neurology and senior author of the study, said in a press release.
Next steps may include the implementation of AI-based models in regular clinical practice to allow physicians to more quickly assess the effects of treatments on their patients, and in clinical trials to allow researchers to test new therapies more safely and effectively, using smaller groups of patients.
“In conclusion, this study demonstrates the value of modeling routine imaging data with high-dimensional [AI-based] techniques that closely reflect the complexity of the brain, not only as a tool for illuminating diseases mechanisms, but as a practical, real-world means of determining the power of therapeutic interventions to change them, both for individual patient care and interventional studies,” the researchers wrote.