Machine Learning of Walking Patterns Could Help Predict MS Progression
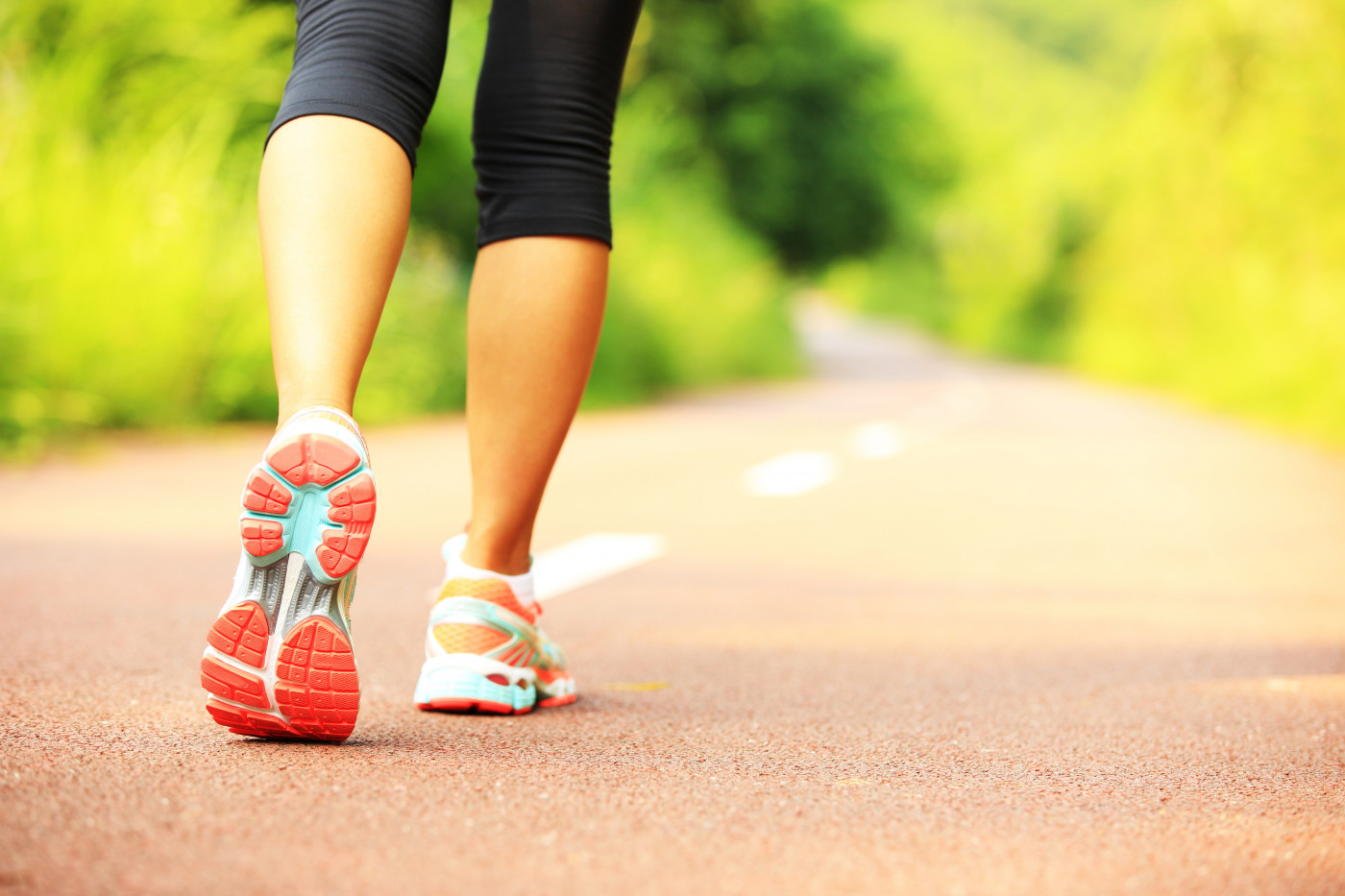
A machine learning-based method that examines walking abnormalities in people with multiple sclerosis (MS) could help identify patients who are at high risk of worsening symptoms, a study suggests.
The study, “Predicting Multiple Sclerosis from Gait Dynamics Using an Instrumented Treadmill – A Machine Learning Approach,” was published in Institute of Electrical and Electronics Engineers Transactions on Biomedical Engineering.
Difficulty walking is one of the most common symptoms of MS. However, walking abnormalities can be difficult to measure objectively — each person’s gait is distinct, as it is affected by the particular mechanics of their body. Plus, walking patterns tend to change with age, and it can be difficult to distinguish age-related changes from disease-driven abnormalities in older people with MS.
In the new study, a team led by researchers at the University of Illinois used machine learning to better identify walking abnormalities in older MS patients.
“Biomechanical systems, such as walking, are poorly modeled systems, making it difficult to spot problems in a clinical setting,” Richard Sowers, PhD, professor at the University of Illinois and co-author of the study, said in a press release.
“We wanted to get a sense of the interactions between aging and concurrent MS disease-related changes, and whether we can also differentiate between the two in older adults with MS,” added Manuel Hernandez, PhD, study co-author and also a professor at the university.
Conceptually, machine learning involves feeding a set of data into a computer. Then, based on certain pre-determined mathematical constraints, the computer develops algorithms to make sense of the data (e.g., finding algorithms to distinguish between persons with or without MS).
The researchers collected gait data on 20 older people with MS (average age 61 years), as well as 20 people without MS who were matched to the MS patients in terms of age, weight, and sex. The participants were twice analyzed while walking on a specially-designed treadmill, once while walking, and once while walking and talking simultaneously.
Using specialized software, the researchers collected a battery of variables related to the participants’ gait, such as how quickly they walked, how long each step was, how much space was between their feet. They also used various statistical methods to mathematically account for expected person-to-person differences (for example, taller people usually have longer legs and, consequently, take longer steps).
The results revealed some broad trends in walking patterns. For example, people with MS tended to walk slower, take shorter steps, and have more space between their feet. Importantly, however, these were considered trends and not distinct features of MS.
“In general, no individual or combination of features exhibit clear non-overlapping patterns characterizing MS,” the researchers wrote.
Then, these varied data were fed to computers for machine learning.
“Machine-learning techniques seem to work particularly well at spotting complex hidden changes in performance,” Hernandez said. “We hypothesized that these analysis techniques might also be useful in predicting sudden gait changes in persons with MS.”
A variety of different machine learning algorithms were used. Generally, they showed good performance — for example, many algorithms were able to differentiate between people with or without MS with high accuracy.
Of note, removing pieces of the data made these models less accurate, highlighting the importance of including all of the different aspects of gait that were analyzed.
Among the MS patients, many of the analyzed gait features were significantly correlated with the extent of disability; for example, slower walking speed and shorter step length were significantly associated with higher scores (more disability) on the Expanded Disability Status Scale (EDSS).
Overall, the results “may aid neurologists to understand advancing disease with aging and identify meaningful [machine learning]-based strategies for identifying [people with MS],” the researchers wrote.
The team noted that this kind of gait-based analysis could make it easier to identify people with MS who are about to experience a worsening of symptoms. “Early detection of these inflection points in older [people with MS] may lead to concise and effective detection strategies and in turn benefit both patients as well as clinicians to curtail MS therapy expenses,” they wrote.
A notable limitation of this study is the relatively small number of people analyzed. As such, further research is needed to more fully characterize gait abnormalities among people with MS.
“In this study, we are trying to extract conclusions from data sets that include many measurements of each individual, but a small number of individuals,” Sowers said. “The results of this study make significant headway in the area of clinical machine learning-based disease-prediction strategies.”