AI algorithms may predict cognitive decline in MS over coming year
Models rely on annual processing speed tests that can be used in clinics
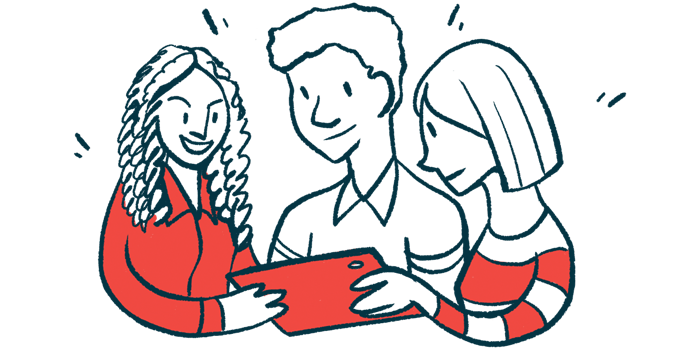
Researchers have developed computer algorithms that may be able to predict certain aspects of cognitive change in multiple sclerosis (MS) patients.
The prediction models, constructed using data from an electronic, self-administered test of information processing speed given MS patients, might accurately identify those likely to experience cognitive worsening over a one-year period.
“If this degree of accuracy is further confirmed with longer follow-ups, the ability to foresee future cognitive deterioration in a specific MS patient could become a reality in clinical practice,” the scientists wrote.
A later start with high efficacy disease-modifying therapies (DMTs) also was seen to be of “top importance” in all predictive models of likely cognitive loss.
Electronic MS cognitive test of information processing speed used
The study, “Predictive models of Multiple Sclerosis-related cognitive performance using routine clinical practice predictors,” was published in Multiple Sclerosis and Related Disorders.
Cognitive dysfunction can be a common MS symptom, affecting up to half of all patients. It can have a significant impact on life, limiting a person’s ability to participate in work and other activities.
However, it is difficult for doctors to adequately assess cognition during routine appointments, mostly because the necessary assessments are time consuming. As such, large and long-term datasets on cognitive trajectories and risk factors for cognitive decline in MS are lacking, the study’s researchers noted.
Computer-based self-assessments offer a way to help overcome these limitations. They allow for the collection of much more data, which can be used to develop machine learning algorithms for predicting cognitive decline.
Machine learning is a type of artificial intelligence in which an algorithm learns to predict certain outcomes from large amounts of data. While often investigated for its utility in predicting MS severity and progression, it’s less frequently used to look at cognition.
Scientists at university hospitals in Spain aimed to develop feasible machine learning models, using measures obtained in routine clinical practice, to predict cognitive evolution in MS.
As part of an ongoing longitudinal study since 2019, they gave an annual electronic-based information processing speed test (PST) Â monitor cognitive function in MS patients at three Madrid clinics.
This test is taken on a tablet and designed to be similar to the Symbol Digit Modalities Test (SDMT), an evaluation of information processing speed used in the clinic.
Study findings then were used to develop a machine learning algorithm for predicting cognitive performance.
Data were analyzed from 1,184 MS patients with a one-year PST follow-up assessment after their initial test. Overall, 187 patients (15.8%) saw a worsening in PST performance at the one-year mark, defined as a reduction of at least 10% on raw processing speed test scores. The remaining majority of patients were determined to have stable cognitive function.
People who experienced cognitive decline were older, had been living with MS longer, had lower starting (baseline) cognitive function, worse baseline disability, and were more likely to have a progressive disease course. They also tended to start treatment with high efficacy DMTs later in their disease course, the researchers noted.
Data from 70% of participants were used to develop machine learning algorithms for predicting cognitive worsening or stability, which were then validated in the remaining 30% of patients.
Predictive accuracy of two models reported to be about 90%
Of six learning models tested, two were identified as having the highest predictive accuracy, at around 90%.
Given the broad demographic and clinical differences between patients who worsened and those who did not, no particular feature was generally considered more important than others at predicting a person’s cognitive outcomes.
The timing of high-efficacy DMT initiation, however, was identified as one of the most important predictors across the machine learning models.
“This result confirms that any predictive model of MS-related cognitive evolution must account for the effect of disease-modifying therapies on cognition, precisely a measure that reflects the [high-efficacy DMT] timing related to the disease onset,” the researchers wrote.
Other factors that may have contributed included baseline cognitive performance, age at first PST, and disease duration.
“Our results demonstrate the possibility of predicting cognitive worsening using routine clinical predictors,” the researchers wrote.
The team noted that the study was limited by the relatively short patient follow-up time, which should be addressed in future long-term studies, as well as the use of only one type of cognitive test. Certain clinical data related to co-existing conditions, relapses, and brain lesions also were lacking.