New AI algorithm provides better MRI images of brain in MS: Study
Fewer scans needed to get 3D image showing brain changes due to disease
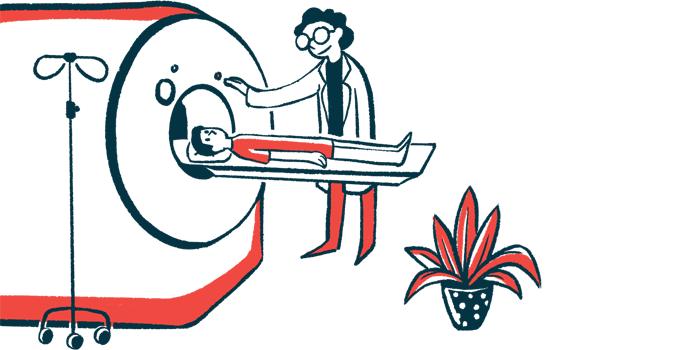
DeepSTI, a new artificial intelligence or AI-assisted MRI algorithm, can generate high-resolution, 3D images of the brain from only one head orientation. This new technology may allow clinicians to use fewer scans to visualize changes in the brain caused by neurological diseases, such as multiple sclerosis (MS), a study reported.
That’s in contrast to a current method, called susceptibility tensor imaging, or STI, that requires multiple scans at different head orientations — making it difficult to use in a clinical setting. DeepSTI instead generates a so-called super-scan of the brain with fewer images, making the process faster and easier for patients.
“Usually, STI imaging requires at least six different scans at different head orientations to achieve a good reconstruction, and that’s mainly why it’s not currently broadly used despite its potential to understand the human brain,” Jeremias Sulam, PhD, the study’s senior author at Johns Hopkins University, in Maryland, said in a university press release.
“Our AI-assisted reconstructions greatly expand the amount of useful information that can be gleaned while requiring much less data, and we hope that will help move this imaging technique from lab to clinic,” Sulam said.
The study, “WaveSep: A Flexible Wavelet-Based Approach for Source Separation in Susceptibility Imaging,” was published in the journal Machine Learning in Clinical Neuroimaging.
Researchers hope to move imaging technique from lab to clinic
In MS, an abnormal immune response causes inflammation and damage to the myelin sheath, a fatty protective coating around nerve fibers, in the brain and spinal cord. Myelin loss results in progressive nerve fiber degeneration and a range of MS symptoms.
MRI scans are used to detect changes to myelin and nerve fibers, referred to as lesions, which can be used to diagnose MS and monitor disease progression and the impact of treatments. The technique uses a strong magnetic field and radio waves to measure water content in tissues, which is then used to create images.
STI is a specialized MRI technique that assesses the magnetic susceptibility of different tissues in the brain by measuring how they become magnetized by the MRI’s magnetic field. It can provide three-dimensional or 3D images of the brain to reveal myelin damage, as well as processes associated with other neurodegenerative diseases like Alzheimer’s disease.
However, because STI requires several MRI scans using different head orientations to get the needed images of the brain, it hasn’t been widely used in the clinic.
The new DeepSTI algorithm takes data from MRI scans and creates a high-resolution, three-dimensional map of magnetic susceptibility in the brain. According to researchers, its key advance is the ability to measure brain tissue such as myelin using fewer scans than previously needed.
Applying machine learning, DeepSTI employs a mathematical method called regularization to provide more accurate reconstitutions of the brain. Essentially, it helps the model focus on generating brain reconstructions that closely resemble what is expected based on prior scan data, enhancing the accuracy and reliability of the results.
“The algorithm is very good at ‘learning’ what brain images should look like,” said Zhenghan Fang, a biomedical engineering graduate student at Johns Hopkins.
“When we run the algorithm on raw data, it uses those found parameters to reconstruct a clearer and more comprehensive image of the brain,” Fang said.
This is a true research success story because not only could it lead to new technologies to aid decision-making in the clinic, but also is opening up new research avenues in more general restoration and reconstruction algorithms and applied mathematics.
The ultimate goal is to make STI a more accessible imaging option for clinicians by reducing the time it takes to perform a scan and enhancing the quality of the image. Sulam has referred to the math powering the algorithm, as “very beautiful.”
“Our team is excited about the mathematical framework that underpins the results here,” Sulam said.
“We’ve been able to answer hard questions that we couldn’t answer before,” Sulam added.
With the data, models, and code now available for other researchers, the team plans to investigate how the algorithm could address other challenging science and engineering problems.
“This is a true research success story because not only could it lead to new technologies to aid decision-making in the clinic, but also is opening up new research avenues in more general restoration and reconstruction algorithms and applied mathematics,” Sulam said.