Machine System Based on Brain Activity May Restore Speech to Those Who Lost It, Study Reports
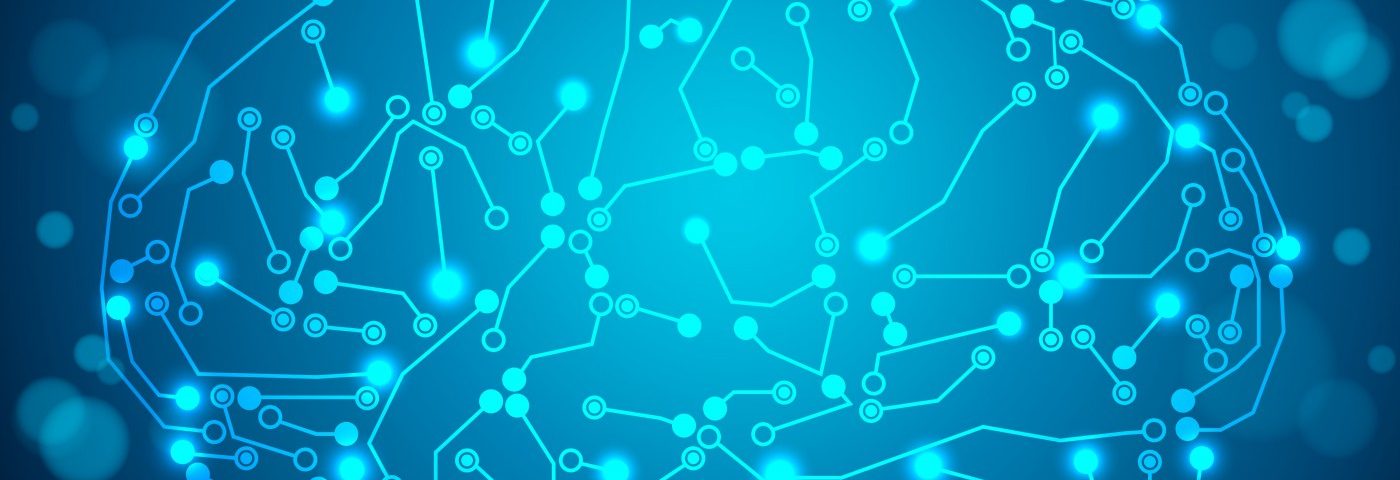
A study suggests that it may be possible to create synthetic speech based on recordings of brain activity in people with irreversible speech loss due to neurological ills, such as multiple sclerosis (MS), Parkinson’s, or stroke.
The system uses tiny electrodes implanted on the surface of the brain that directly records activity that controls speech, and feed that information into a brain-machine interface to generate natural-sounding speech.
The study, led by researchers at University of California San Francisco (UCSF), is titled “Speech synthesis from neural decoding of spoken sentences,” and published in the journal Nature.
According to the team, this new system represents a major advancement over existing technologies: namely, assistive devices that track very small eye or facial muscle movements to generate text or synthesized speech.
The world-renowned scientist Stephen Hawking, for instance, used an infrared switch mounted on his eyeglasses to detect when he moved his cheek. When a cursor moving through a keyboard displayed on his computer screen reached a desired word, Hawking stop it with a twitch of his cheek.
But this is a laborious, error-prone, and slow process, typically permitting a maximum of 10 words per minute, compared to the 100 to 150 words per minute of natural speech.
This newly developed technology detects the specific brain activity that controls the nearly 100 muscles which continuously move the lips, jaw, tongue, and throat to form words and sentences, and feeds this information to a virtual vocal tract (an anatomically detailed computer simulation). A synthesizer then converts these vocal tract movements into a synthetic human voice.
It is being developed in the laboratory of Edward Chang, MD, a neurosurgeon at the UCSF Epilepsy Center and member of the UCSF Weill Institute for Neuroscience.
“For the first time, this study demonstrates that we can generate entire spoken sentences based on an individual’s brain activity,” Chang said in a UCSF news release written by Nicholas Weiler. “This is an exhilarating proof of principle that with technology that is already within reach, we should be able to build a device that is clinically viable in patients with speech loss.”
Chang specializes in surgeries to remove brain tissue in patients with severe epilepsy, and who do not respond to medications. To prepare for these operations, neurosurgeons place high-density arrays of tiny electrodes onto the surface of the patients’ brains, a technique called electrocorticography or ECoG.
ECoG helps surgeons pinpoint the specific brain area triggering patients’ seizures, and also allows them to map out key areas, such as those involved in language, they want to avoid damaging.
Chang and colleagues used ECoG to record the brain’s electrical activity in people reading aloud several hundred natural sentences, and used the information collected to construct a map of those brain areas that control specific parts of the vocal tract. Of note, the persons analyzed were five volunteers with intact speech being treated at UCSF Epilepsy Center.
The team then used linguistic principles to determine which vocal cord, tongue, or lip movements were needed to produce specific sounds in the spoken sentences.
Researchers then created a “virtual vocal tract,” consisting of a decoder that transformed brain activity patterns produced during speech into movements of the virtual vocal tract, and a synthesizer that converted these vocal tract movements into a synthetic voice.
A YouTube video included in the news article helped to illustrate the synthetic voice is understandable. Researchers put it to the test by recruiting hundreds of listeners through Amazon Mechanical Turk (MTurk), an internet crowdsourcing marketplace.
These transcribers accurately identified 69 percent of synthesized words from lists of 25 alternatives, and transcribed 43 percent of sentences with perfect accuracy. When the list of alternatives was increased to 50 words to choose from, the overall accuracy dropped to 47 percent, but listeners were still able to understand 21 percent of synthesized sentences perfectly.
“We still have ways to go to perfectly mimic spoken language,” said Josh Chartier, a bioengineering graduate student in the Chang lab and study co-author. “We’re quite good at synthesizing slower speech sounds like ‘sh’ and ‘z’ as well as maintaining the rhythms and intonations of speech and the speaker’s gender and identity, but some of the more abrupt sounds like ‘b’s and ‘p’s get a bit fuzzy. Still, the levels of accuracy we produced here would be an amazing improvement in real-time communication compared to what’s currently available.”
The team is now testing higher-density electrode arrays, and more advanced machine learning algorithms to further improve the synthesized speech.
Although the study was conducted in volunteers with normal speaking ability, its researchers believe their approach could one day restore a voice to people who have lost the ability to speak due to neurological damage, much as robotic limbs controlled by the brain restore movement.
“Decoded articulatory representations were highly conserved across speakers, enabling a component of the decoder to be transferrable across participants,” the study concluded. “Furthermore, the decoder could synthesize speech when a participant silently mimed sentences. These findings advance the clinical viability of using speech neuroprosthetic technology to restore spoken communication.”