Machine learning model predicts CIS to MS conversion risk: Study
Researchers say model is first to use MRI, clinical, demographic data
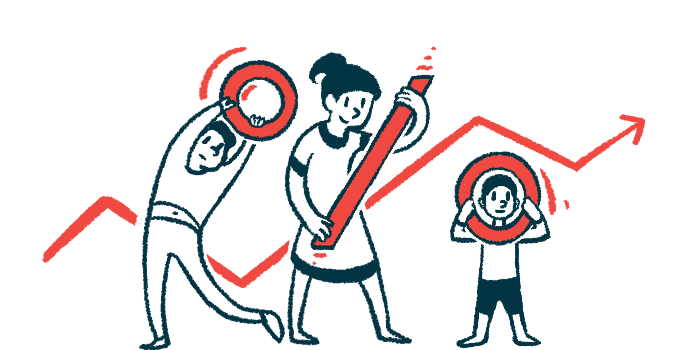
A new machine learning model is able to predict the risk of converting from clinically isolated syndrome, or a first event of multiple sclerosis (MS)-like symptoms, to clinically definite disease, a study found.
“Our study developed a machine-learning model that not only provides a numerical estimate of the risk of a patient with CIS developing [clinically definite MS] but also offers sufficient interpretability to understand the basis of its predictions,” its researchers wrote.
The study, “Predicting the conversion from clinically isolated syndrome to multiple sclerosis: An explainable machine learning approach,” was published in Multiple Sclerosis and Related Disorders.
In most patients, MS is marked by relapses or flares in which symptoms suddenly worsen, followed by periods of remission where symptoms ease or disappear entirely. In order to confirm a clinical diagnosis of relapsing MS, the person has to experience more than one such attack, or have signs that brain damage has occurred at multiple points in time. (Hence the “multiple” in “multiple sclerosis.”)
Someone who has experienced only one MS-like attack and has no other signs of MS is said to have clinically isolated syndrome (CIS). If someone with CIS experiences subsequent attacks or had evidence of new brain damage, that person may be diagnosed with definite MS. But not everyone with CIS goes on to develop MS.
Predicting CIS to MS conversion poses challenges
Recent studies have shown that giving MS treatments to people with CIS can reduce the risk of developing MS, but these medications are costly and carry risks of side effects.
In theory, it would be best to give more potent treatments to people with CIS who are at highest risk of converting to clinically definite MS, while patients with lower risk might receive safer, lower-efficacy therapies or wait for treatment. Accurately predicting the risk of MS in people with CIS has proven a challenge, however.
A team of scientists in Iran created a machine learning model to predict the risk of MS in CIS patients based on a combination of clinical and demographic data.
“To the best of our knowledge, this study is the first to develop a [machine learning] model that utilizes a combination of MRI characteristics, clinical data, and demographic information to estimate the probability of CIS converting to [clinically definite] MS,” the scientists wrote.
In machine learning models, a computer is trained to use pre-established mathematical rules or algorithms to identify patterns in complex data. By identifying these patterns, the computer learns how to make sense of future data by looking for similar patterns.
To create their model, the researchers used a dataset of 273 CIS patients in Mexico who were followed over the course of a decade, during which time 46% developed clinically definite MS. The scientists used data from most of the patients to train the model, then tested its accuracy using data from the remaining patients.
This revealed promising accuracy: In the test, the model was able to accurately identify 75% of CIS patients who went on to develop MS and 81% of those who did not.
Training helps model identify variables
Although machine learning models can be useful tools, a common drawback is that it can be difficult to identify which factor(s) are most important in the model after training. To address this, the researchers conducted a series of experiments where they retrained the model using different combinations of variables, looking to see which types of data were needed for the computer to make accurate predictions.
“This approach enhances the interpretability of the model, providing a clear understanding of how each variable influences the prediction,” the researchers wrote. “This is particularly important in medical applications, where understanding the reasoning behind a prediction can be as crucial as the prediction itself.”
This identified nine key factors, including demographic factors such as age, gender, and education, as well as certain features on MRI imaging and specific symptoms.
“These findings highlight the multifactorial nature of [MS] progression and the importance of considering a broad range of variables in predictive models,” the researchers wrote.
The team said that further studies using larger datasets will be needed to validate the machine learning model.