New AI tool accurately detects transition from RRMS to SPMS
Use of artificial intelligence may be predictive vs. retrospective: Study
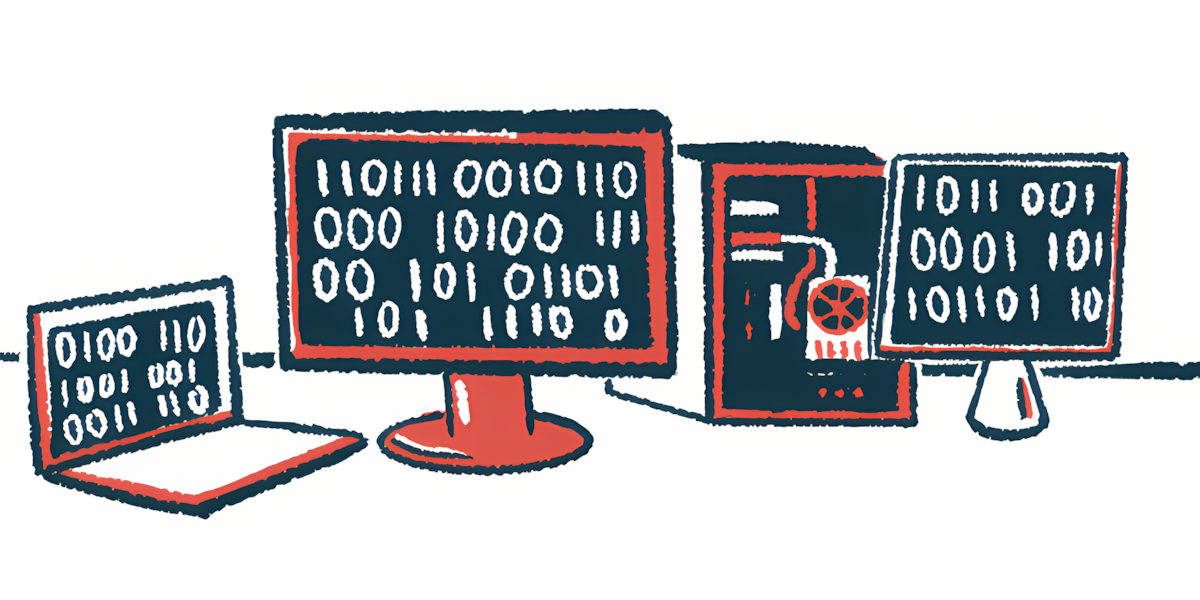
A new machine learning tool, a form of artificial intelligence (AI), may accurately detect the transition from relapsing-remitting multiple sclerosis (RRMS) to secondary progressive multiple sclerosis (SPMS) — and may be able to do so even earlier than clinicians can — a new study showed.
According to the researchers, their newly developed AI model may improve diagnostics in multiple sclerosis (MS).
“By recognising patterns from previous patients, the model can determine whether a patient has the relapsing-remitting form or whether the disease has transitioned to secondary progressive MS,” Kim Kultima, PhD, the study’s senior author and a professor at Uppsala University in Sweden, said in a university news story.
Per the study team: “We present here a first-of-its-kind predictive model that is able to distinguish between RRMS and SPMS at high accuracy.”
The team noted that “the gradual transition” among patients from RRMS to SPMS “is often diagnosed retrospectively with a typical delay of three years.” This new model has the potential “to distinguish between RRMS and SPMS at each individual [doctor] visit,” the researchers wrote.
The study “Conformal prediction enables disease course prediction and allows individualized diagnostic uncertainty in multiple sclerosis,” was published in the journal npj Digital Medicine.
Researchers use AI tool to develop predictive model for MS
Most people with multiple sclerosis initially develop RRMS, which is marked by relapses, where symptoms worsen, interspersed with periods of remission when symptoms ease. Over time, many people with RRMS will transition to SPMS — characterized by symptoms that get gradually worse over time irrespective of relapse activity.
Diagnosing the transition from RRMS to SPMS is important because these two MS types have distinct responses to the disease-modifying therapies now available to patients.
“Proactive recognition of patients with progressive disease could limit exposure to ineffective medications and their side effects,” the researchers wrote.
To date, however, there are no solid criteria defining the transition between disease types. Usually such diagnoses are made by looking at how a patient’s symptoms have changed from previous years. As such, patients generally are not diagnosed with SPMS until they’ve already been living with this type of MS for several years.
To improve MS diagnoses, Kultima and colleagues set out to create a machine learning tool to detect the transition from RRMS to SPMS based on data collected from patients at routine checkups.
Machine learning is a form of AI that uses mathematical rules called algorithms to analyze a large set of data, learn from its analyses, and make predictions based on the learned patterns.
To build the model, the researchers used data from more than 22,000 people with MS, collected between 1972 and 2022, in the Swedish MS Registry.
Data from most of the patients was used to train, validate, and calibrate the machine learning tool, and data from the remaining 3,595 patients was used to subsequently test it. The test dataset comprised 2,451 people with RRMS, 677 with SPMS, and 467 who had an initial RRMS diagnosis but then transitioned to SPMS during follow-up.
In 26% of cases, model ID’d MS transition earlier than doctors
The results showed that the new model overall was 92.1% accurate at discriminating between RRMS and SPMS at a given hospital visit. The model also correctly predicted an initial RRMS diagnosis and a later transition to SPMS in 68.5% of the 467 patients who experienced such transition.
In more than half of these cases, the tool identified the switch from RRMS to SPMS at almost exactly the same time as patients’ records changed. Moreover, in 26.6% of cases, the model identified the switch at an earlier hospital visit than doctors had.
“For patients, this means that the diagnosis can be made earlier, which makes it possible to adjust the patient’s treatment in time and slow down the progression of the disease,” Kultima said. “This also reduces the risk of patients receiving medicines that are no longer effective.”
What is unique about the model is that it also indicates how confident it is in each individual assessment. … This means that the doctor will know how reliable the conclusion is and how confident the AI is in its assessment.
In addition to identifying which type of MS the patient has, the machine learning tool includes conformal prediction — basically, a way for the computer to report how certain it is that its labeling is correct for each patient.
“What is unique about the model is that it also indicates how confident it is in each individual assessment,” Kultima said. “This means that the doctor will know how reliable the conclusion is and how confident the AI is in its assessment.”
The researchers have made a version of their tool publicly available online so that other researchers and clinicians can explore its utility.
“In the long term, the model could also be used to identify suitable participants for clinical trials – which could contribute to more effective and individualised treatment strategies,” Kultima said.